AI/ML on Kubernetes: Deploying Models with Pulumi on Google Cloud
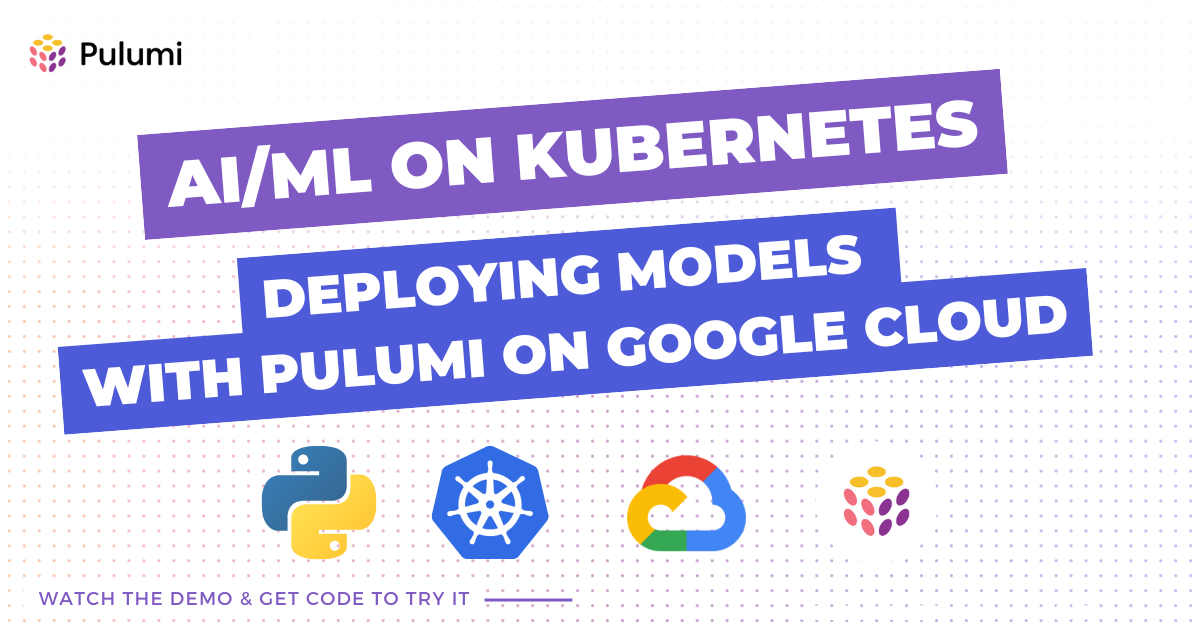
Kubernetes has transformed cloud infrastructure by enabling scalable, containerized applications. While it initially gained traction for managing web applications and microservices, its capabilities now extend to AI/ML workloads, making it the go-to platform for data scientists and machine learning engineers.
Running AI/ML workloads on Kubernetes presents unique challenges, including:
- Specialized hardware requirements (e.g., GPUs, TPUs)
- Scalability for model training and inference
- Complex data pipelines that integrate various cloud services
- Infrastructure automation for seamless deployment
Google Cloud Kubernetes (GKE) provides a robust foundation for AI/ML workloads, but managing infrastructure manually can be cumbersome. This is where Pulumi comes in—enabling Infrastructure as Code (IaC) to automate and simplify AI/ML infrastructure on Kubernetes.